基准测试¶
介绍¶
研发能力的基准测试是该领域一个重要的研究问题。我们不断探索基准测试这些能力的方法。当前的基准测试列在此页面上。
开发能力基准测试¶
基准测试用于评估固定数据因素的有效性。它主要包括以下步骤:
配置¶
- pydantic settings rdagent.components.benchmark.conf.BenchmarkSettings¶
Show JSON schema
{ "title": "BenchmarkSettings", "type": "object", "properties": { "bench_data_path": { "default": "example.json", "format": "path", "title": "Bench Data Path", "type": "string" }, "bench_test_round": { "default": 10, "title": "Bench Test Round", "type": "integer" }, "bench_test_case_n": { "anyOf": [ { "type": "integer" }, { "type": "null" } ], "default": null, "title": "Bench Test Case N" }, "bench_method_cls": { "default": "rdagent.components.coder.factor_coder.FactorCoSTEER", "title": "Bench Method Cls", "type": "string" }, "bench_method_extra_kwargs": { "additionalProperties": true, "title": "Bench Method Extra Kwargs", "type": "object" }, "bench_result_path": { "default": "result", "format": "path", "title": "Bench Result Path", "type": "string" } }, "additionalProperties": false }
- 配置:
env_prefix: str = BENCHMARK_
- 字段:
- field bench_data_path: Path = PosixPath('example.json')¶
基准测试数据
- field bench_method_cls: str = 'rdagent.components.coder.factor_coder.FactorCoSTEER'¶
测试用例使用的方法
- field bench_method_extra_kwargs: dict [Optional]¶
除任务列表外,待测试方法的额外关键字参数
- field bench_result_path: Path = PosixPath('result')¶
结果保存路径
- field bench_test_case_n: int | None = None¶
要运行的测试用例数量;如果未给出,则将运行所有测试用例
- field bench_test_round: int = 10¶
要运行的轮数,每轮可能耗时10分钟
示例¶
bench_test_round
的默认值为10,运行大约需要2小时。要将其从 10
修改为 2
,请按照以下所示调整 .env 文件中的环境变量。
BENCHMARK_BENCH_TEST_ROUND=2
数据格式¶
bench_data_path
中的示例数据是一个字典,每个键代表一个因素名称。与每个键相关联的值是包含以下信息的因素数据:
描述:因素的文本描述。
公式:表示模型公式的 LaTeX 公式。
变量:涉及该因素的变量字典。
类别:因素的类别或分类。
难度:实现或理解该因素的难度级别。
gt_code:与该因素相关联的一段代码。
以下是该数据格式的示例:
{
"Turnover_Rate_Factor": {
"description": "A traditional factor based on 20-day average turnover rate, adjusted for market capitalization, which is further improved by applying the information distribution theory.",
"formulation": "\\text{Adjusted Turnover Rate} = \\frac{\\text{mean}(20\\text{-day turnover rate})}{\\text{Market Capitalization}}",
"variables": {
"20-day turnover rate": "Average turnover rate over the past 20 days.",
"Market Capitalization": "Total market value of a company's outstanding shares."
},
"Category": "Fundamentals",
"Difficulty": "Easy",
"gt_code": "import pandas as pd\n\ndata_f = pd.read_hdf('daily_f.h5')\n\ndata = data_f.reset_index()\nwindow_size = 20\n\nnominator=data.groupby('instrument')[['TurnoverRate_30D']].rolling(window=window_size).mean().reset_index(0, drop=True)\n# transfer to series\nnew=nominator['TurnoverRate_30D']\ndata['Turnover_Rate_Factor']=new/data['TradableACapital']\n\n# set the datetime and instrument as index and drop the original index\nresult=pd.DataFrame(data['Turnover_Rate_Factor']).set_index(data_f.index)\n\n# transfer the result to series\nresult=result['Turnover_Rate_Factor']\nresult.to_hdf(\"result.h5\", key=\"data\")"
},
"PctTurn20": {
"description": "A factor representing the percentage change in turnover rate over the past 20 trading days, market-value neutralized.",
"formulation": "\\text{PctTurn20} = \\frac{1}{N} \\sum_{i=1}^{N} \\left( \\frac{\\text{Turnover}_{i, t} - \\text{Turnover}_{i, t-20}}{\\text{Turnover}_{i, t-20}} \\right)",
"variables": {
"N": "Number of stocks in the market.",
"Turnover_{i, t}": "Turnover of stock i at day t.",
"Turnover_{i, t-20}": "Turnover of stock i at day t-20."
},
"Category": "Volume&Price",
"Difficulty": "Medium",
"gt_code": "import pandas as pd\nfrom statsmodels import api as sm\n\ndef fill_mean(s: pd.Series) -> pd.Series:\n return s.fillna(s.mean()).fillna(0.0)\n\ndef market_value_neutralize(s: pd.Series, mv: pd.Series) -> pd.Series:\n s = s.groupby(\"datetime\", group_keys=False).apply(fill_mean)\n mv = mv.groupby(\"datetime\", group_keys=False).apply(fill_mean)\n\n df_f = mv.to_frame(\"MarketValue\")\n df_f[\"const\"] = 1\n X = df_f[[\"MarketValue\", \"const\"]]\n\n # Perform the Ordinary Least Squares (OLS) regression\n model = sm.OLS(s, X)\n results = model.fit()\n\n # Calculate the residuals\n df_f[\"residual\"] = results.resid\n df_f[\"norm_resi\"] = df_f.groupby(level=\"datetime\", group_keys=False)[\"residual\"].apply(\n lambda x: (x - x.mean()) / x.std(),\n )\n return df_f[\"norm_resi\"]\n\n\n# get_turnover\ndf_pv = pd.read_hdf(\"daily_pv.h5\", key=\"data\")\ndf_f = pd.read_hdf(\"daily_f.h5\", key=\"data\")\nturnover = df_pv[\"$money\"] / df_f[\"TradableMarketValue\"]\n\nf = turnover.groupby(\"instrument\").pct_change(periods=20)\n\nf_neutralized = market_value_neutralize(f, df_f[\"TradableMarketValue\"])\n\nf_neutralized.to_hdf(\"result.h5\", key=\"data\")"
},
"PB_ROE": {
"description": "Constructed using the ranking difference between PB and ROE, with PB and ROE replacing original PB and ROE to obtain reconstructed factor values.",
"formulation": "\\text{rank}(PB\\_t) - rank(ROE_t)",
"variables": {
"\\text{rank}(PB_t)": "Ranking PB on cross-section at time t.",
"\\text{rank}(ROE_t)": "Ranking single-quarter ROE on cross-section at time t."
},
"Category": "High-Frequency",
"Difficulty": "Hard",
"gt_code": "#!/usr/bin/env python\n\nimport pandas as pd\n\ndata_f = pd.read_hdf('daily_f.h5')\n\ndata = data_f.reset_index()\n\n# Calculate the rank of PB and ROE\ndata['PB_rank'] = data.groupby('datetime')['B/P'].rank()\ndata['ROE_rank'] = data.groupby('datetime')['ROE'].rank()\n\n# Calculate the difference between the ranks\ndata['PB_ROE'] = data['PB_rank'] - data['ROE_rank']\n\n# set the datetime and instrument as index and drop the original index\nresult=pd.DataFrame(data['PB_ROE']).set_index(data_f.index)\n\n# transfer the result to series\nresult=result['PB_ROE']\nresult.to_hdf(\"result.h5\", key=\"data\")"
}
}
确保数据放置在 FACTOR_COSTEER_SETTINGS.data_folder_debug
中。数据文件应为 .h5
或 .md
格式,并且不得存储在任何子文件夹中。LLM-Agents 将审核文件内容并执行任务。
运行基准测试¶
完成 安装与配置 后启动基准测试。
dotenv run -- python rdagent/app/benchmark/factor/eval.py
完成后,将生成一个 pkl 文件,其路径将在控制台的最后一行打印。
显示结果¶
analysis.py
脚本从 pkl 文件读取数据并将其转换为图像。请修改 rdagent/app/quant_factor_benchmark/analysis.py
中的 Python 代码,以指定 pkl 文件的路径和 png 文件的输出路径。
dotenv run -- python rdagent/app/benchmark/factor/analysis.py <log/path to.pkl>
一个 png 文件将被保存到指定路径,如下所示。
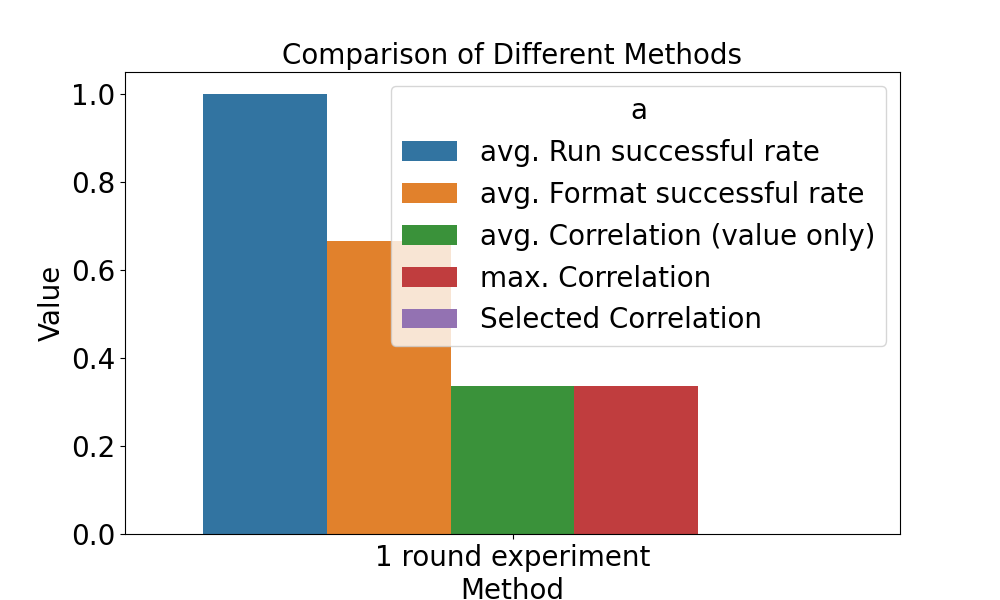